30under30 Nominee Brina Seidel
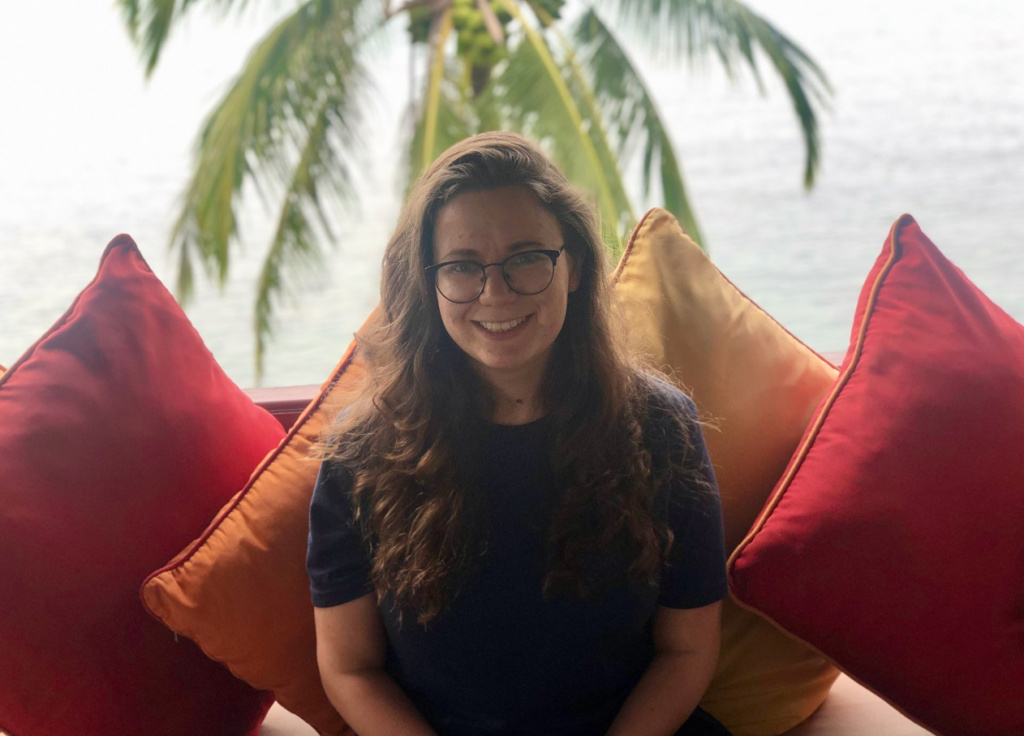
We interviewed Significant Insights Global 30 Under 30 2022 Nominee Brina Seidel, a Staff Data Scientist at Premise working on machine learning models that bring companies in-store insights about their products and their brand.
So, how did you get into the industry, and take us through how you got to this point?
I took a pretty circuitous route into the industry. I started my career doing research on economic policy and international development. At that time, I was using econometrics to study trends in global poverty and foreign aid.
Eventually, I realized that my favorite part of my job was simply answering questions with data. The issues I had been working on were interesting and important, but I wasn’t exactly going to be the one to end global poverty and I knew my data skills could have a more immediate impact in another sector. I went to grad school, transitioned into data science, and eventually made my way to Premise.
At Premise, my job is to extract insights from the images collected through our app. Premise collects data for customers in a variety of sectors, but I quickly found that our commercial customers had the most immediate use for advanced image analytics. I built out a set of computer vision models that extract information from pictures of products on the shelf in grocery stores, and that process has led me into the market research space. Being a data scientist isn’t just about knowing machine learning — you also really have to understand how the data is created and how your model predictions will be used. In this case, that meant I had to learn about how shelves are organized and stocked, what determines product placement, what metrics manufacturers and distributors care about, and more. I’ve really loved learning about the market research space and I’m excited to see what other data science applications emerge in this field.
Career paths are rarely without challenges. Can you share an honest moment from your career when things didn’t go quite according to plan, but the lessons remain with you to this day?
Absolutely. In my first data science role, I spent a lot of time building models for a product that didn’t exist yet. The models were performing well on historical data offline and, in a vacuum, they sounded like a good idea. Everyone seemed to agree that this work was a good use of time. But I should have stepped back and thought seriously about the problems we were trying to solve. If I’d done so, I would have seen that there was a long list of things that would have been far more useful for our customer than the stuff I was working on.
I learned a lot about following the wrong signals in your career from that experience. Even if executives respond really well to your powerpoint presentation, you may not be on the right track. You have to be honest with yourself about the impact you’re having.
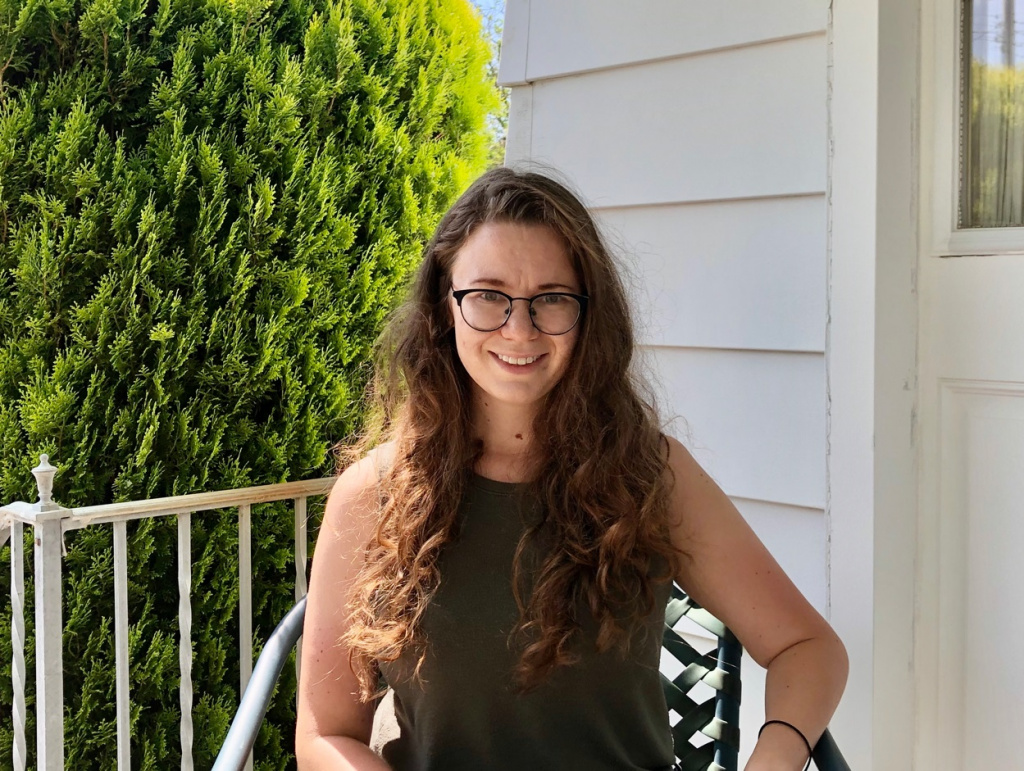
What two things should junior researchers focus on as they progress in their careers?
For junior researchers using data science techniques, I think it’s really important to find the right balance between 1) methods that are clean and simple and 2) methods that are exciting and cutting-edge.
The field is flush with new and exciting methodologies that are just plain cool — but they’re often not the right tool for the job. There’s a real temptation to spend your time (and compute resources!) on state-of-the-art deep learning even when more traditional methods could give you results that are just as good for a fraction of the cost and complexity. There’s something really beautiful about taking a complex question and distilling it into something that can be answered with a short query or a couple lines of matrix math. At the same time, it really is important to stay up to date with new research so that you can spot the cool methods that are truly useful as soon as they emerge. I do my best to read academic papers and experiment with new open-source models so that I understand the range of available options.
At the end of the day, succeeding as a data scientist is about building models that can be put into production to solve real problems and provide real insights. If you keep that goal in mind, you’ll be able to strike the right balance.
How do we ensure that students and those leaving school aspire to join our sector?
I said earlier that I ended up in this sector simply because I like answering questions with data — and I think there are a lot of students out there who like the same thing. Our job is to show them that market research questions are really interesting!
I also think it’s crucial to help students, and young women in particular, understand that technical roles in this field are an option for them. The best data scientists I know come from non-traditional backgrounds. Once you have some foundational skills in statistics and data wrangling, you just need the drive to keep learning about data science. That means asking subject matter experts useful questions about your data, experimenting with techniques you’ve never tried before, and doing a whole lot of googling. If that sounds like something you can do, it’s worth giving data science a try.
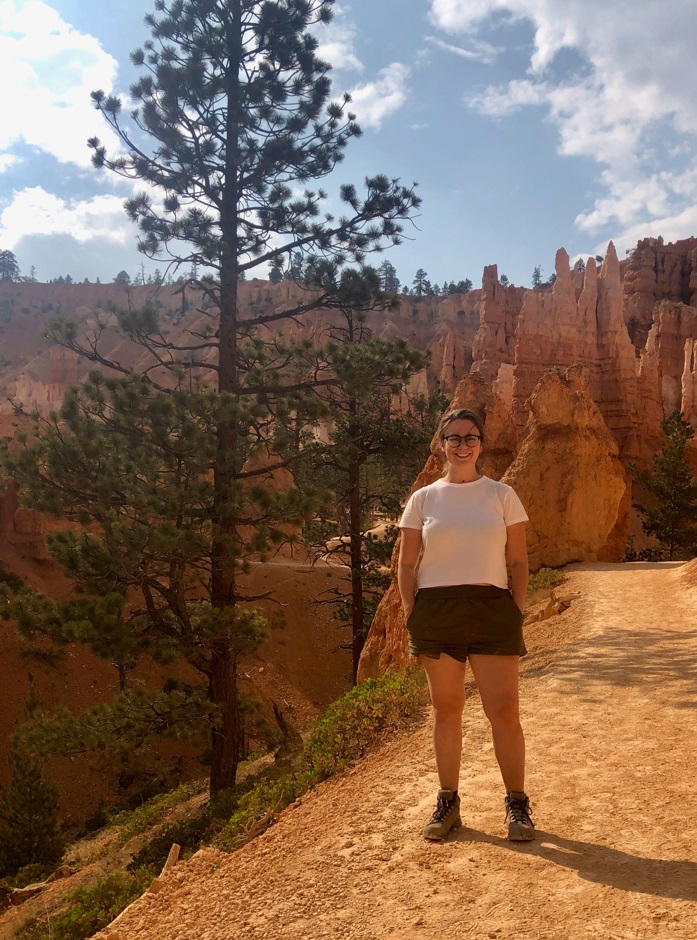
Do you have any advice for our sector?
It’s such an exciting time to explore ways to use machine learning to understand what consumers buy. Deep learning research has been progressing by leaps and bounds for the past decade, but we’ve only recently started seeing those advancements brought to bear in applied settings like market research. My best advice is to continually search for new sources of data and new ways to analyze the data you already have, because novel ideas are emerging at an unprecedented rate.
And do you have anyone who has helped your career so far that you’d like to acknowledge and say thanks or give a shout out to?
Of course! I’ve been lucky enough to work with incredible people at every stage of my career. Most recently, I learned some important lessons from my old manager Tim about how to do practical, impactful data science at a startup. The whole team at Premise has taught me so much and I’m grateful for the opportunities I’ve had here.